 |
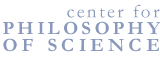
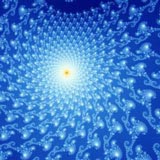

home
::: about
::: news
::: links
::: giving
::: contact

events
::: calendar
::: lunchtime
::: annual
lecture series
::: conferences

people
::: visiting fellows
::: postdoc fellows
::: senior fellows
::: resident fellows
::: associates

joining
::: visiting fellowships
::: postdoc fellowships
::: senior fellowships
::: resident fellowships
::: associateships

being here
::: visiting
::: the last donut
::: photo album
|
 |
 |
 |
2010
Clark Glymour, Philosophy of Computation and Causation
Theme of the Lectures: How Computers Can (Help) Discover Causation
Lecture 1: Could there be computer algorithms for scientific discovery?
Many famous philosophers, scientists and statisticians claimed that no
algorithms--no computerized procedures--are possible for discovering
scientific truths. Einstein maintained that scientific theories are a
"free creation"; Karl Popper denied that there really is a "logic of
scientific discovery"; Carl Hempel maintained that innovations in
scientific theory require "novel predicates" that computers cannot
invent. I will argue that all of these arguments are fallacious and
give examples of discovery by computer from economics, ecology,
neuroscience, epidemiology and elsewhere. Further, I claim that new
methods of acquiring data require discovery by computer.
Lecture 2: Causal relations for computation.
Causal descriptions in ordinary and scientific language are rich and
varied. To develop algorithms for discovering causal relations we must
represent causal relations by definite mathematical objects. Moreover,
the mathematical objects must imply features that can be measured and
used to test or search for the causal relations that best explain our
data. I will introduce directed graphical representations of causal
relations and their probabalistic properties--sometimes called "causal
Bayes nets."
Lecture 3: Algorithms for Discovery
This lecture will introduce two discovery algorithms and explain how they
work and the conditions under which they have been proved correct.
Examples will be given from ecology and elsewhere.
Lecture 4: Discovery in Difficult Cases
This lecture will review some of the many ways that data may mislead us
about causal relations, and describe some of the algorithms for dealing
with such problems. Illustrative applications will be given, but I will
not go into the details of the algorithms.
Lecture 5: Neuroscience, an illustration
|
 |